National contact
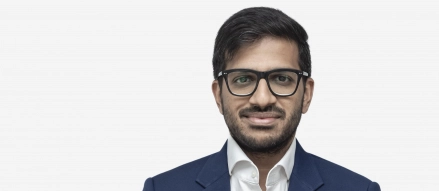
As banks are expanding their use of data to deliver meaningful insights, ensuring this data is of the right quality is vital. However, consistently achieving high quality data remains a challenge, with clean-up and remediation projects only providing immediate to short-term quality assurance. Banks, therefore, need a more long-term and integrated approach to ensure data quality issues are promptly identified, reported to appropriate individuals and then monitored throughout.
In developing this comprehensive data quality approach, banks should consider the following:
The IT architecture of most banks present a picture of defragmented data sources subject to inconsistent taxonomies and technical rules. As data flows across systems, the threat to data quality grows - data attributes may drop off or data may be altered and become unsuitable for further use. This increases reliance on manual intervention and tactical solutions to reconcile, adjust and update the data.
Banks should therefore focus on harmonising their IT infrastructure and developing a single source of truth. This will provide a reliable and efficient way for pulling out, aggregating and transforming different types of data required for various regular or ad-hoc purposes.
According to the April 2020 Basel Committee report on progress towards implementing BCBS 239 ‘Principles for Effective Risk Data Aggregation and Risk Reporting’, good progress has been made in implementing strong governance arrangements. This includes having a clearer definition of roles and responsibilities, setting up governance committees, establishing enterprise data strategies and performing more independent validation of data management and implementation efforts.
Banks should continue their efforts in strengthening the oversight framework and implementing this framework in a consistent manner across geographies and business activities. In addition, with the growing involvement of third parties in data related processes, banks should ensure that their arrangements for overseeing third parties cover data management aspects.
As today’s world sees banks address a myriad of changes (be it restructuring business activities, changing operating model or complying with additional regulatory requirements), data, taxonomies, dictionaries and transformation capabilities must be updated to reflect these changes.
Banks should consider automated, streamlined and traceable maintenance procedures as well as a clear handshake between data management roles to ensure these updates are implemented. Furthermore, quality controls throughout the data lifecycle should be robust enough to flag when maintenance procedures have not been performed accurately and timely.
In conclusion, banks need to take a long and holistic view to data quality. There has to be a shift away from implementing quick fixes to deep diving and addressing root causes of data quality gaps.
Building the structures that support a sustainable data quality programme should not be handled in isolation, but rather integrated into enterprise-wide technology projects and risk management efforts.
For more information or support in implementing sound data quality practices, please get in touch.
References
This website uses cookies.
Some of these cookies are necessary, while others help us analyse our traffic, serve advertising and deliver customised experiences for you.
For more information on the cookies we use, please refer to our Privacy Policy.
This website cannot function properly without these cookies.
Analytical cookies help us enhance our website by collecting information on its usage.
We use marketing cookies to increase the relevancy of our advertising campaigns.